Transformative Technology Development
Multimodal mass spectrometry imaging of mouse and human liver
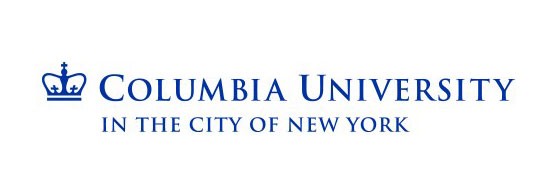
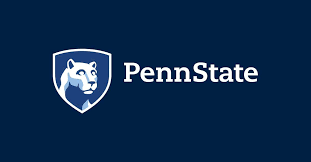
This group is working to develop a multimodal mass spectrometry imaging pipeline with novel desorption sources and data integration that will enable cell-type specific multi-omic profiling in 3-dimensions at high spatial resolution (micron to submicron) and high speed (>10 ms/pixel) in froze-hydrated biological tissues at single cell level. This would provide previously inaccessible information on cellular and tissue organization, and on how homeostasis and disease intersect at the level of different cells types within the tissue.
A major challenge for performing multi-omics using mass spectrometry imaging has been the (i) lack of universal ionization methods, (ii) limited sample preparation protocols for preserving pristine chemical gradients, (iii) low sensitivity, (iv) integration of different omics in the same sample and at the single cell level, and (v) limited tools for integration of large quantities of data. These laboratories are developing systematic mass spectrometry imaging (MSI) for high sensitivity and high resolution analysis of diverse tissues. They discovered that water-based gas cluster ion beams (H2O-GCIB) operating at high energy yield ionization enhancements of multiple biomolecules (e.g., metabolites, lipids, and peptides/protein fragments) with high sensitivity at 1 µm lateral resolution and without labeling or complicated sample preparation. Coupled with unique buncher-ToF Secondary Ion Mass Spectrometry (SIMS) instrumentation and cryogenic sample handling, they have imaged biomolecules directly in cells and tissues at near-native state (i.e., frozen-hydration) with feature resolution of 1-10 µm. Low concentration biomolecules (e.g., cardiolipin and metabolites) that were impossible to localize in single cells previously are now visible with 3-dimensional localization. Moreover, with sufficient signal per pixel, they can use automated data analysis to characterize biologically active cell types, cellular metabolic pathways, multilevel heterogeneities and functional sites within 1 µm2 and areas of interest (up to 20 cm2). They further developed software algorithm to combine multimodal imaging data from the same and adjacent sections to create integrated spatial multiomics with single cell resolution.
The labs will develop a pipeline for MSI analysis of biomolecules, and to elucidate molecular heterogeneity in tissues using multimodal imaging. To support the multi-modal analysis pipeline, they will develop an integrated data analysis platform to automate the processing of large data sets. Integration of multi-omics remains challenging, particularly spatially localizing multiple biomolecules at single cell level. The direct visualization of cellular contents provides information on biomolecular composition, interactions, pathways and functions. This network of biomolecules is the driving force of specific behavior of cells in physiological states. Despite this, a comprehensive grasp of these interactions at cellular level has not moved beyond segregated methods. These efforts will result in an integrated multimodal imaging platform to summon the best characteristics of each image form, acquiring a complete picture of the biomolecular network at spatial resolution of 1 µm. With this direct visualization, they will address how lipidomics and metabolism links with functional biomarkers that stem from metabolism-associated protein complexes and phase-separated membrane-less organelles at the subcellular level, and how this drives different cell functions, cell death modalities, including different modes of cell death.
Fast Facts
Project title: | Multimodal mass spectrometry imaging of mouse and human liver |
Organ specialty: | Liver |
PIs: | Brent Stockwell, Columbia, and Hua Tian, University of Pittsburgh |
Co-Investigator: | Nicholas Winograd, Penn State |
Project Manager: | Hua Tian, University of Pittsburgh |
Assay types: | DESI, GCIB-SIMS, spatial transcriptomics |
Grant number: | 1UG3CA256962-01 |
Learn more: |
Visit the Columbia lab website, follow Brent on Twitter, follow Hua on Twitter. |